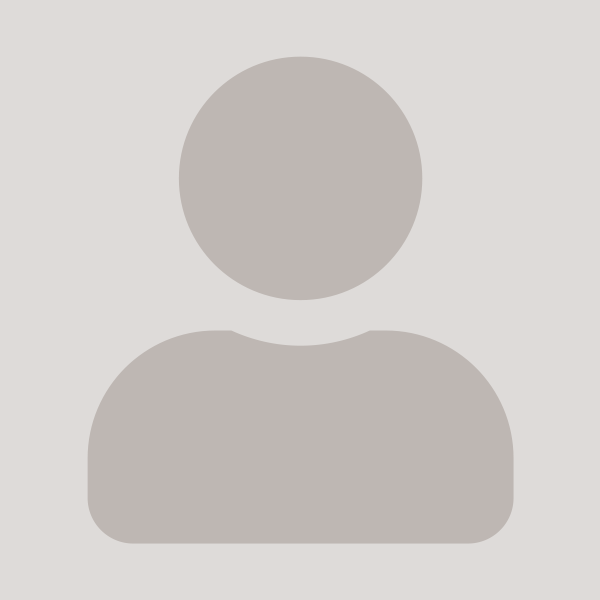
Bo Bernhardsson
Modellering och styrning av osäkra system. Programdirektör för masterprogrammet i maskininlärning, system och styrning.
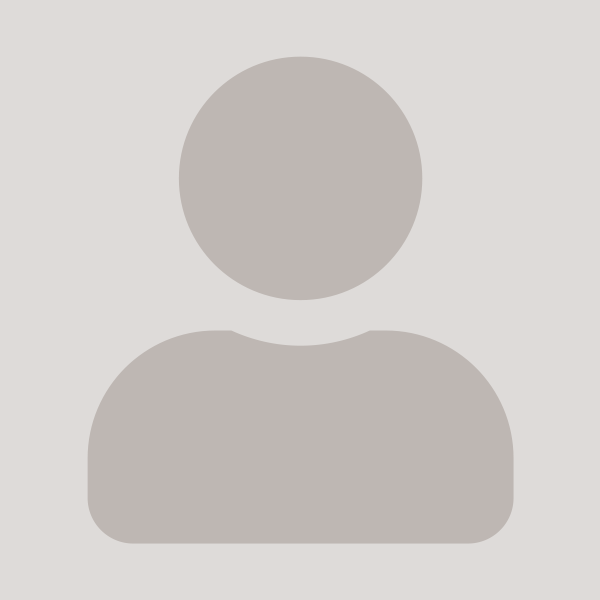
Model-free MIMO control tuning of a chiller process using reinforcement learning
Författare
Summary, in English
The performance of HVAC equipment, including chillers, is continuing to be pushed to theoretical limits, which impacts the necessity for advanced control logic to operate them efficiently and robustly. At the same time, their architectures are becoming more complex; many systems have multiple compressors, expansion devices, evaporators, circuits, or other elements that challenge control design and resulting performance. In order to maintain respectful controlled speed of response, stability, and robustness, controllers are becoming more complex, including the move from thermostatic control, to proportional integrator (PI), and to multiple-input multiple-output (MIMO) controllers. Model-based control design works well for their synthesis, while having accurate models for numerous product variants is unrealistic, often leading to very conservative designs. To address this, we propose and demonstrate a learning-based control tuner that supports the design of MIMO decoupling PI controllers using online information to adapt controller coefficients from an initial guess during commissioning or operation. The approach is tested on a physics-based model of a water-cooled screw chiller. The method is able to find a controller that performs better than a nominal controller (two single PI controllers) in terms of decreasing deviations from the operating point during disturbances while still following reference changes.
Avdelning/ar
- Institutionen för reglerteknik
- LTH profilområde: AI och digitalisering
- LTH profilområde: Teknik för hälsa
Publiceringsår
2023
Språk
Engelska
Sidor
782-794
Publikation/Tidskrift/Serie
Science and Technology for the Built Environment
Volym
29
Issue
8
Dokumenttyp
Artikel i tidskrift
Förlag
Taylor & Francis
Ämne
- Engineering and Technology
- Control Engineering
Status
Published
Projekt
- Efficient Learning of Dynamical Systems
ISBN/ISSN/Övrigt
- ISSN: 2374-4731