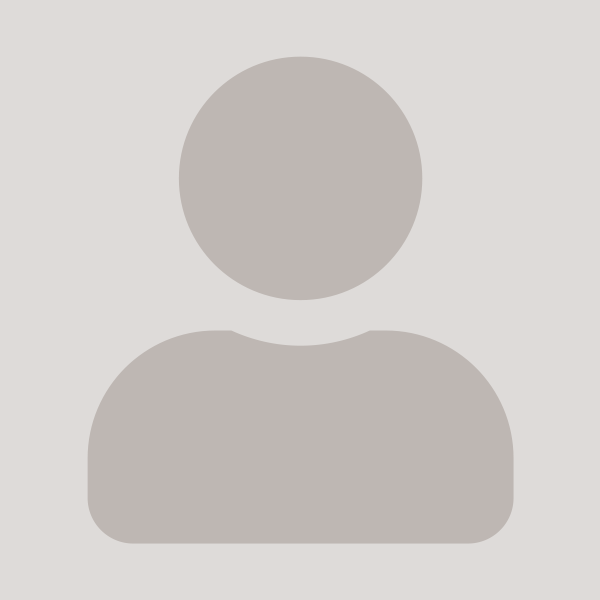
Bo Bernhardsson
Modellering och styrning av osäkra system. Programdirektör för masterprogrammet i maskininlärning, system och styrning.
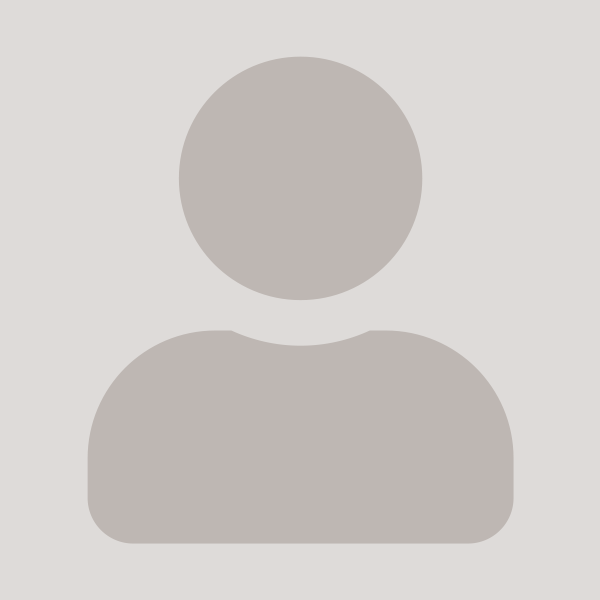
Improving EEG-based decoding of the locus of auditory attention through domain adaptation
Författare
Summary, in English
Avdelning/ar
- LU profilområde: Naturlig och artificiell kognition
- LTH profilområde: AI och digitalisering
- LTH profilområde: Teknik för hälsa
- Institutionen för reglerteknik
- ELLIIT: the Linköping-Lund initiative on IT and mobile communication
Publiceringsår
2023
Språk
Engelska
Publikation/Tidskrift/Serie
Journal of Neural Engineering
Dokumenttyp
Artikel i tidskrift
Förlag
IOP Publishing
Ämne
- Control Engineering
Status
Published
Projekt
- Optimizing the Next Generation Brain Computer Interfaces using Cloud Computing
ISBN/ISSN/Övrigt
- ISSN: 1741-2560